Yieldmo Achieves 15% Increase in Click-through-Rate with Kumo AI Predictions
October 10, 2024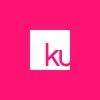
Kumo team
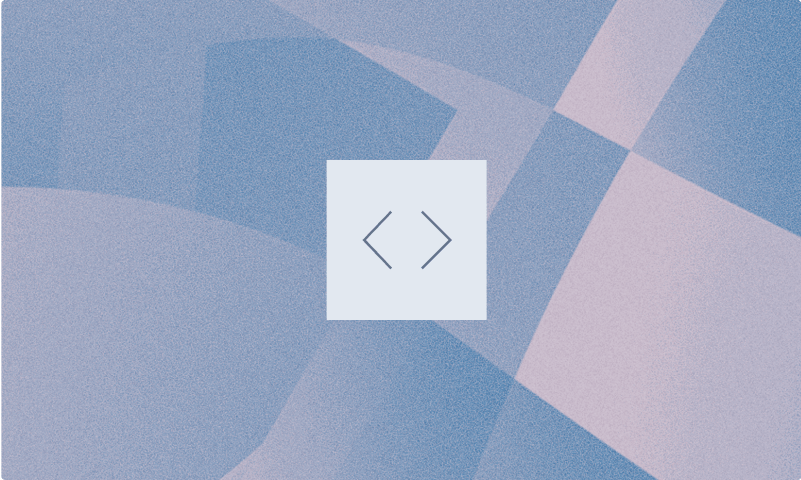
Impact:
- 20% improvement in targeting accuracy by mapping relationships between users and URLs
- 15% increase in ad relevance by predicting the likelihood of interactions between different entities
- 5-10% increase in downstream model performance using Kumo embeddings to improve the quality of input data for secondary models
- 15% increase in campaign KPIs like Click-Through-Rate
- Days saved by automating much of the feature engineering process
Introduction
Yieldmo is a digital advertising platform that helps brands invent creative experiences through tech and AI, using bespoke ad formats, proprietary attention signals, predictive format selection, and privacy-safe premium inventory curation. Yieldmo believes all ads should be human-centered, tailored, and provoke users’ emotions and actions. Yieldmo helps brands deliver the best ad for every impression opportunity, merging creative and media for proven results.
With the digital advertising industry moving away from third-party cookies due to increasing privacy concerns and regulatory changes, companies are seeking alternative methods to reach their target audiences. Major web browsers, such as Google Chrome and Apple Safari, are phasing out support for third-party cookies to enhance user privacy and data protection. This shift requires advertisers and digital platforms to adopt new strategies and technologies for audience targeting as ad buys are increasingly likely to rely on ID-independent targeting solutions.
Yieldmo partners with Kumo AI to improve audience targeting and enhance ad performance. Offering a solution that could seamlessly integrate with Yieldmo’s existing infrastructure, which included Snowflake as its central data warehouse, various data processing pipelines, and multiple ad-serving platforms, Kumo provided the necessary tools to improve ad relevance and effectiveness while navigating the transition away from third-party cookie-based audience targeting.
This solution would ultimately need to take the form of an “audience recommendation system” wherein Yieldmo could input a series of known users, and output a recommended set of targetable assets (URLs, placements) that were not dependent on cookie-based targeting.
Scalability and Precision Challenges in Ad Targeting
Before implementing Kumo, Yieldmo faced challenges inherent to digital advertising operations:
- Scaling Recommendation Systems: As Yieldmo’s user base and ad inventory grew, their existing recommendation systems required efficient ways for scaling. Yieldmo captures dozens of signals on billions of impressions per day using their patented Attention signal measurement system, and combines that with Environmental signals from the bid stream to drive performance lift for their customers. While they had access to sophisticated algorithms, the complexity and volume of their data required a more advanced solution. Traditional recommendation engines were not equipped to handle the extensive and intricate relationships within their data, particularly with the need for personalized and context-aware recommendations. Kumo’s graph transformer architecture provided the necessary sophistication to maintain accuracy and relevance in ad targeting, significantly improving their recommendation system’s performance.
- Need for Personalized and Context-Aware Recommendations: Yieldmo needed to deliver personalized and context-aware recommendations to enhance user engagement and ad performance. However, traditional recommendation models could not fully capture the complexity of user behavior and preferences as they lacked the ability to understand rich contextual data, and relied on simple user attributes and past interactions. Additionally, these models required extensive manual feature engineering, which was time-consuming and inflexible, and they could not integrate and analyze diverse data sources effectively.
- Addressing the Decline of Third-Party Cookies and the Need for Innovative Targeting Methods: With the industry moving away from third-party cookies due to increased privacy concerns, Yieldmo had to find new ways to accurately target individuals based on user behavior, without relying on outside data sources. Over 50% of impressions are already cookieless, and that number is expected to grow. Although Google officially delayed the deprecation of the third-party cookie, the train has already left the station and it’s essential for advertisers to garner the efficient reach available in traditionally non-addressable advertising impression opportunities.
These challenges highlighted the need for an advanced AI solution that could not only handle the increased data volume and complexity but also provide more accurate, personalized, and context-aware recommendations. This is where Kumo’s innovative AI technology came into play, providing Yieldmo with the tools needed to overcome these obstacles and revolutionize its ad targeting strategy.
Solution
Kumo AI provided Yieldmo with a comprehensive solution to address its ad targeting challenges. Here’s how Kumo’s capabilities transformed Yieldmo’s operations:
- Using AI to Create Predictive ML Models: Kumo introduced sophisticated graph transformer architecture and link prediction models to enhance Yieldmo’s recommendation systems. These models effectively handled the complexity and scale of Yieldmo’s data, enabling more accurate and context-aware ad targeting. By mapping relationships between users and URLs, Kumo improved targeting accuracy by 20%.
- Integration with Snowflake: The integration of Kumo AI with Yieldmo’s existing infrastructure was designed to be seamless and efficient. Kumo’s platform was configured easily with Yieldmo’s Snowflake data warehouse and existing data pipelines, minimizing the need for manual intervention. This integration process allowed Yieldmo to quickly leverage Kumo’s advanced predictive AI capabilities, ensuring optimal performance and immediate impact on their audience recommendation system.
- Specific Features and Capabilities of Kumo’s Technology: Yieldmo leveraged several key features of Kumo’s AI platform:
- Graph Transformer Architecture: Kumo effectively handled the complexity and scale of Yieldmo’s data by mapping relationships between users and URLs. This improved model accuracy by 20%.
- Link Prediction Models: These models enabled Yieldmo to predict the likelihood of interactions between different entities within their data, enhancing the relevance of audience recommendations.
- Embeddings: Kumo generated embeddings that provided a deeper understanding of user behavior and preferences. This contributed to a 5-10% increase in downstream model performance by improving the quality of input data for secondary models.
- Eliminating Feature Engineering:
- Kumo automated much of the feature engineering process, significantly reducing the time and effort required from Yieldmo’s data science team. This automation enhanced the quality of the features used in the models, leading to a 5-10% improvement in downstream model performance.
By leveraging these advanced AI capabilities, Yieldmo overcame the scalability, computational, and precision challenges it faced, setting the stage for improved ad performance and more effective audience targeting.
Results and Impact
The integration of Kumo AI’s capabilities yielded significant improvements in Yieldmo’s ad targeting and performance metrics:
- 20% Accuracy Improvement in Link Prediction: “Tests showed that Kumo’s link prediction models outperformed previous methods, leading to a 15% increase in campaign KPIs (like Click-Through-Rate).” This meant that ads were more effectively reaching the right audience, driving higher interactions and conversions.
- 5-10% Improvement in Downstream Models: “The secondary models trained on Kumo’s outputs saw a consistent 5-10% improvement in performance.”
- Efficient Data Processing: By leveraging Kumo’s advanced AI tools, Yieldmo was able to process and analyze large datasets more efficiently, ensuring timely and accurate predictions.
- Significant Time Savings in Feature Engineering: Feature engineering is often an iterative and time-consuming process that requires deep analysis of all features, and in the case of Yieldmo, involves thousands of features. “Kumo’s ability to handle feature engineering drastically reduced the time spent on this task, allowing the data science team to focus on more strategic initiatives.”
Future Plans
Building on the success of the initial integration, Yieldmo plans to expand the application of Kumo AI to other areas of its platform:
- Further Enhancing Audience Targeting: Yieldmo aims to continue improving its audience targeting algorithms by leveraging Kumo AI to refine and optimize its ad placement strategies further. This will allow Yieldmo to deliver more relevant and personalized ads, enhancing user engagement and campaign performance.
- Exploring New Use Cases: Yieldmo is looking to explore new use cases for Kumo’s advanced AI tools. “We are excited to see how Kumo’s AI can help us enhance user engagement metrics and personalize ad content more effectively.” This includes leveraging Kumo’s capabilities to understand and predict user behavior better, leading to improved ad targeting and performance.
- Continuous Improvement and Optimization: Yieldmo plans to work closely with Kumo to continuously monitor and optimize its AI models. “Regular collaboration with Kumo will ensure our models remain at the cutting edge of ad technology and maintain high-performance standards.” This ongoing partnership will help Yieldmo stay ahead in the competitive ad tech landscape.
- Integration with Additional Data Sources: Yieldmo intends to integrate additional data sources into its AI models to provide even more comprehensive and accurate predictions. By incorporating a wider range of data, Yieldmo can enhance the precision and effectiveness of its ad targeting strategies, leading to better outcomes for advertisers and users alike.
Leveraging Kumo AI, Yieldmo positions itself as competitive in the rapidly evolving digital advertising landscape, ensuring it can continue to deliver high-quality, relevant ads to its audience.
Learn more about Kumo AI by requesting a demo.