Speeding Up Graph Learning Models with PyG and torch.compile
April 28, 2025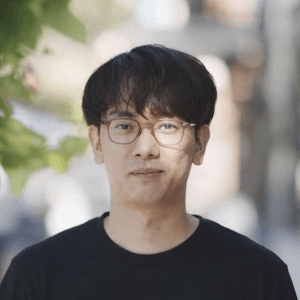
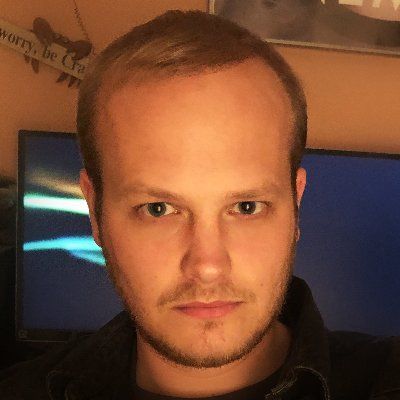
Akihiro Nitta, Matthias Fey
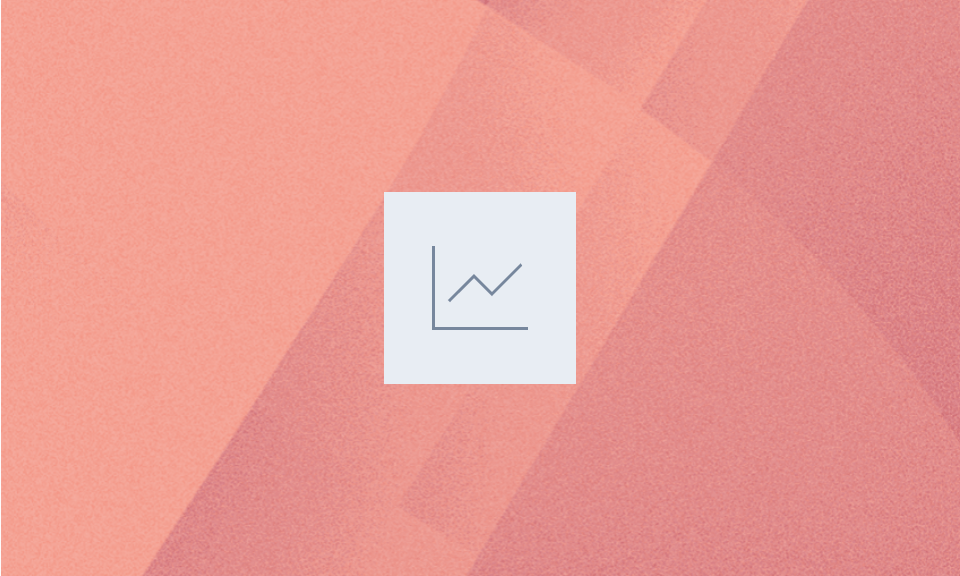
This blog post will guide you on how to speed up your PyTorch graph machine learning models and maximize the potential of torch.compile
and PyTorch. We'll explore practical techniques through our Relational Deep Learning example using PyG, gaining speed improvements of up to 35% in our experiments without sacrificing accuracy.
Behind the Scenes of Relational Deep Learning
Relational Deep Learning (RDL) is an advanced approach in AI that combines deep learning techniques with relational reasoning to model and learn from interconnected, structured data effectively. By leveraging graph-based representations — such as those found in relational databases — RDL enables neural networks to capture and utilize the complex dependencies and interactions between different entities. As we described in our blog post, RDL constructs a large-scale heterogeneous graph from relational tables, where each table corresponds to a node type, and each row of a table is an instance of the node type in the graph, with matched primary key-foreign key pairs defining the edges. At its core, it employs Graph Transformers and Graph Neural Networks (GNNs) to achieve state-of-the-art performance by incorporating connectivity patterns in graphs and diverse multi-modal features in tables.
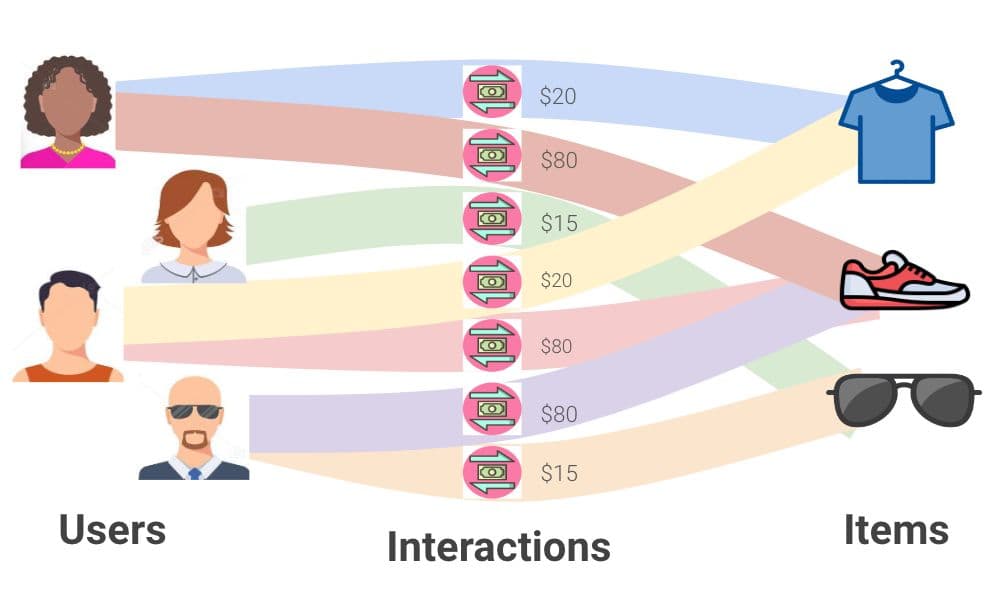
To implement Relational Deep Learning at Kumo, we leverage open-source ML libraries such as PyTorch Geometric (PyG), PyTorch Frame, and PyTorch Lightning, all built on top of PyTorch. PyTorch is our deep learning backend and provides the necessary flexibility with its eager mode to design powerful and complex architectures. On top of these, we leverage PyTorch Frame for multi-modal data processing and PyG for message passing within GNNs and Graph Transformers. Specifically, we first encode multi-modal features — numerical, categorical, multi-categorical, text, images, timestamp, etc. — into a shared embedding space using PyTorch Frame. PyG then uses the encoded features as node features and performs message passing across tables to make predictions, ensuring temporal information is incorporated to avoid data leakage. Finally, PyTorch Lightning is used to structure and scale training processes. It helps us write less boilerplate code and handle different types of graph learning tasks for various use cases such as item recommendation, customer churn prediction, or fraud detection.
Compiling PyTorch Models with torch.compile
PyTorch’s eager mode excels during the development and debugging phase of model design. However, in production, performance — both in terms of speed and memory efficiency — becomes a top priority. With the introduction of PyTorch 2.0, we can leverage torch.compile
, which JIT-compiles PyTorch code into optimized kernels with a simple wrapper:
model = MyModule()
+ model = torch.compile(model)
While torch.compile
offers overall runtime improvements without requiring any code changes, we did notice some slowdowns in certain areas, as well as some existing issues that became more pronounced. For instance, we experienced approximately 20 seconds of latency at the end of each epoch, which was not present when running in eager mode. Additionally, profiling the compiled training steps revealed another slowdown that was not significant in eager mode: the host had to wait for the device to complete specific operations during each training step. Ideally, we expected the host and device to operate asynchronously. In the next section, we will discuss the specific optimizations that enabled us to address these challenges and fully leverage the speed enhancements that torch.compile
can provide.
Maximizing the Benefits of torch.compile
To fully leverage the performance gains of torch.compile
, we needed to dive deep into the specific slowdowns we encountered. In this section, we will break down the key slowdowns we observed, such as blocking host-device synchronizations and unexpected code re-compilations, and will share the practical optimizations that helped us mitigate them.
Avoid Unnecessary Re-Compilations
Whenever torch.compile
sees that an assumption in a region of user code changed, PyTorch re-compiles it with the new assumption. This can take from a few tens of seconds to minutes depending on the model. These assumptions can be in data types, constant values, shapes, etc. With TORCH_LOGS=recompiles
, you can check what triggers these re-compilations. Here, we cover two examples, such as re-compilations due to (1) dynamic shapes and (2) the use of a learning rate scheduler.
(1) By default, PyTorch assumes input shapes don’t change in subsequent iterations, and when it sees a new shape, it re-compiles the code so that the new optimized code can handle different shapes.
model = torch.compile(model)
model(torch.randn(10)) # compiles model for shape `10`
model(torch.randn(11)) # re-compiles it for dynamic shapes
In RDL, input shapes change every iteration because each subgraph has a different number of nodes due to the nature of neighbor sampling and the way how PyG handles sparsity and mini-batching, e.g., each user may have a varying length of order history.
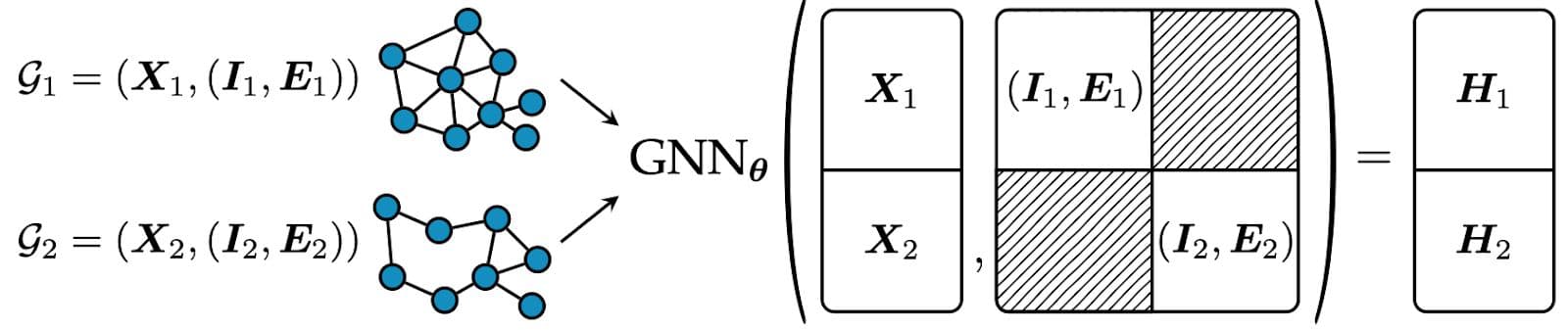
Although PyTorch automatically detects whether input shapes differ across iterations by default as we covered above, we explicitly tell torch.compile
to optimize our code for dynamic shapes from the beginning. We do this instead of optimizing it for static shapes in the first iteration and re-optimizing it for dynamic shapes when it sees a different shape in the second iteration by setting torch.compile(dynamic=True)
:
model = torch.compile(model, dynamic=True)
model(torch.randn(10)) # compiles model for dynamic shapes
model(torch.randn(11)) # triggers no recompilation
(2) Also, we observed that compiling optimizer.step
causes re-compilations whenever a learning scheduler updates the learning rate. It turned out that torch.compile
assumes that the learning rate in the optimizer is a constant value when it’s a float
, and thus, whenever the learning rate scheduler updates the value, torch.compile
’s assumption is invalidated, leading to re-compilation. To avoid this unnecessary recompilation, we had to use a Tensor
as a learning rate per Compiled Optimizer w/ LR Scheduler Now Supported.
- optimizer = torch.optim.Adam(model.parameters(), lr=0.01)
+ optimizer = torch.optim.Adam(model.parameters(), lr=torch.tensor(0.01))
lr_scheduler = torch.optim.lr_scheduler.LinearLR(optimizer, total_iters=5)
Addressing Graph Breaks
When torch.compile
sees some user code that it cannot bake into a computation graph, it sets a “graph break”. Having a graph break means the compiler is unable to fuse kernels that are separated across different graphs, even though fusing would be possible if those operations were captured within the same graph. This leads to more data movement on GPU, which the compiler was trying to eliminate. For the compiler to not miss such optimization opportunities, we addressed graph breaks where possible by looking at logs produced with TORCH_LOGS=graph_breaks
. Usually, setting this environment variable produces helpful messages about the causes of graph breaks (e.g., pytorch/pytorch#111551, pytorch/pytorch#112338). Oftentimes, graph breaks like these are easy to resolve with a few lines of code change:
# Graph break example fixed in
# https://github.com/pyg-team/pytorch_geometric/pull/8363
def forward(self, x: Tensor) -> Tensor:
- key = ("author", "writes", "paper")
+ key = "<author___writes___paper>"
out = self.module_dict[key](x)
...
The above code block shows an example that we observed in PyG. To address the graph break, we had to work around it by passing a key of str
instead of tuple[str, str, str]
to ModuleDict.__getitem__(key)
.
Considering Enabling CUDA Graphs
CUDA Graphs is a feature that allows you to record GPU operations as a graph and replay the graph, enabling multiple CUDA kernels to be executed through a single CPU launch.
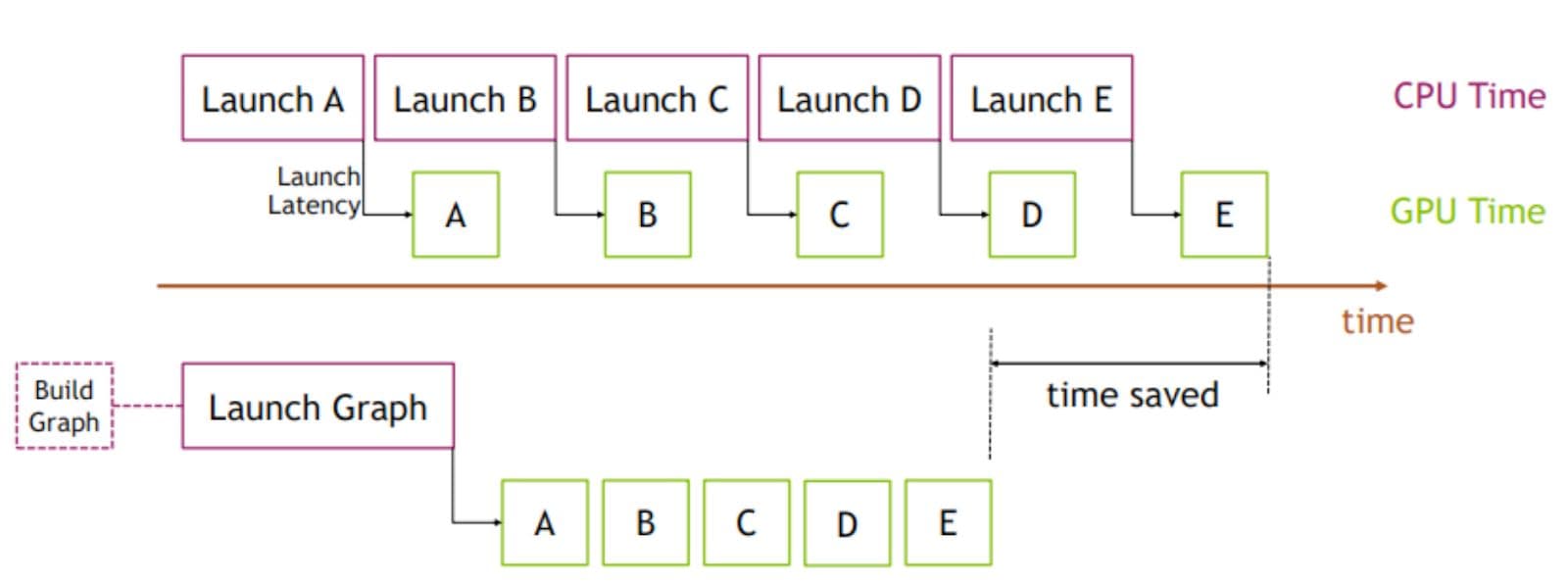
PyTorch supports CUDA Graphs for dynamic shapes via torch.compile(mode='reduce-overhead')
, and there is a discussion on enabling CUDA Graphs by default (pytorch/pytorch#121968). While it is beneficial for static shape cases or dynamic shapes but with a limited variety of shapes, we kept it disabled as the number of nodes in each mini-batch of subgraphs differs across iterations. Otherwise, it becomes unusable due to more memory usage and significant slowdown since it records the kernel launches again in each iteration every time it sees a new shape.
Remove Device Synchronizations
Python runs asynchronously with GPU execution — it launches GPU kernels and continues without waiting for the GPU kernels to complete unless you explicitly or implicitly force a synchronization between CPU and GPU. For example, when you call cuda_tensor.item()
or float(cuda_tensor)
, your Python interpreter has to wait until its data on GPU is transferred to CPU and is blocked from launching subsequent CUDA kernels, leading to the launching overhead — GPU being idle until the subsequent kernels are launched. The following code block shows common examples of producing such a synchronization between CPU and GPU:
# Example 1:
- metric = 0
+ metric = torch.zeros(1, device='cuda').squeeze_()
for step in range(max_steps):
...
- metric += int((label == pred).sum())
+ metric += (label == pred).sum()
# Example 2:
- x = torch.repeat_interleave(x, repeats)
+ x = torch.repeat_interleave(x, repeats, output_size=size)
Example 1 shows a case where it previously forced a device-to-host synchronization in every iteration to accumulate a counter metric during the epoch, and the new code removes the synchronization by accumulating the metric on the device side so that it doesn’t have to transfer it to the host every iteration. A similar issue happened in our case through an old version of torchmetrics
. However, by upgrading torchmetrics
to a version that includes a patch Lightning-AI/torchmetrics#2480, it no longer forces the synchronization.
In Example 2, it is not obvious from the user code why a synchronization gets triggered, however, calling torch.repeat_interleave
without output_size
argument triggers a synchronization in PyTorch because it needs to calculate the output size and allocate the memory of the output tensor before launching the CUDA kernel as documented in the torch.repeat_interleave
doc page.
Following other Optimization Best Practices
We recommend following torch.compile
, the missing manual, the Performance Tuning Guide provided by PyTorch, and Lightning-AI/pytorch-lightning#12398 for other optimization opportunities in PyTorch programs, such as hiding communication latency or data transfer latency behind GPU computation.
Staying Up-To-Date on Latest PyTorch Releases
Staying up-to-date with the latest releases of PyTorch-related packages is highly recommended. For example, we have made updates to PyG to make it more torch.compile
-friendly, and we will continue to do so. This helps to address excessive re-compilations, graph breaks, or synchronizations that such packages produce and to benefit from the latest performance improvements of the compiler.
Experiments
We benchmarked training efficiency improvements by torch.compile
‘ing our graph learning models on Kaggle H&M recommendation challenge dataset across these three tasks:
user-churn
: A node classification task predicting whether a customer will churn in the next week.item-sales
: A node regression task estimating the total sales of an article for the upcoming week.user-item-purchase
: A link prediction task forecasting the set of articles each customer will purchase over the next seven days.
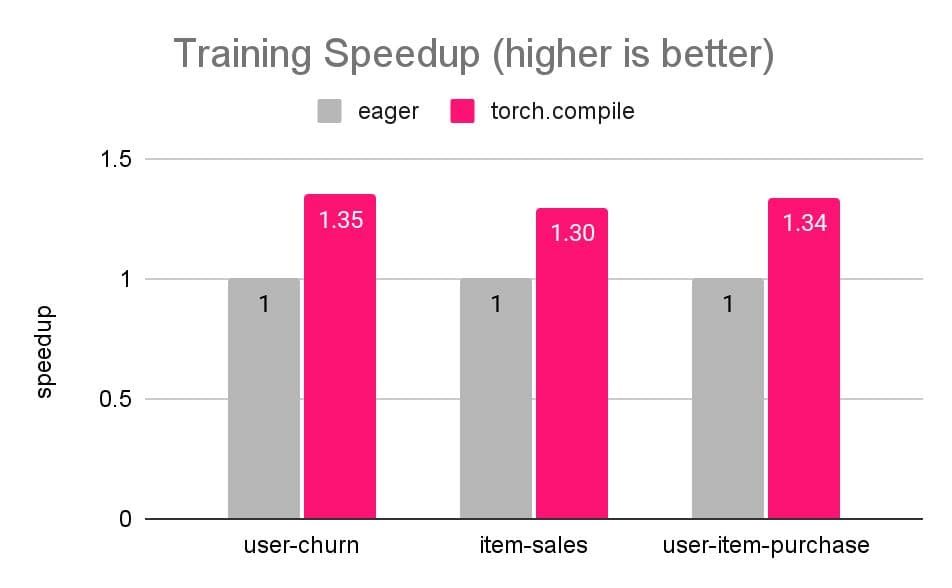
While our models have been developed with efficiency in mind already, we observe that we can improve them further via torch.compile
between 30% to 35% without compromising the predictive accuracy of the models.
Conclusion
While we’ve long relied on PyTorch for its flexibility in research and model design, the advent of torch.compile
has allowed us to push our Graph Transformer and GNN models into production with significant speedups. By tuning for dynamic shapes, resolving graph breaks, avoiding recompilation, and minimizing device-host synchronizations, we’ve unlocked up to a 35% runtime improvement on real-world graph learning tasks. We hope these lessons from scaling training at Kumo can serve as a practical guide for the broader PyTorch community looking to maximize performance with torch.compile
.